Still sailing peacefully with AI, or has the term started irritating you by now?
You might feel the technology is either fine, impressive or perhaps not worth the hype. But it seems the tech world isn’t backing off!
They not only want to show you how great AI is but also aim to push the boundaries even further—who knows what’s next?
One thing is undeniable, the world is embracing more and more data, and with that data, the tech world will train numerous AI models for various purposes.
In the midst of the AI hype, have you noticed how crucial it is to have enough data to train an AI?
And even more importantly, how vital it is to have the right data?
Are we ensuring data quality governance before importing data to AI algorithms?
It’s not that engineers are training AI models on unrefined data, but there are still biases that occur.
Here’s where AI data governance comes into the picture!
This highlights the need for high-quality data and most importantly the right information!
“According to NIST’s Reva Schwartz, the main distinction between the draft and final versions of the publication is the new emphasis on how bias manifests itself not only in AI algorithms and the data used to train them but also in the societal context in which AI systems are used!”
So, what can be done to address this?
The first and most important step is to train AI models on the right, well-analyzed, and unbiased data.
And that’s where data governance comes into play!
But what exactly is data governance?
Let’s explore that!
What is Data Governance?
Data governance is a practice that involves setting some data standards for organizing and managing data to ensure accuracy, security, and compliance.
It includes setting rules and assigning roles to handle data properly.
This approach helps –
- Maintain Data Quality – Ensures information is accurate and reliable.
- Enhance Security – Protects sensitive data from unauthorized access.
- Ensure Compliance – Adheres to legal and regulatory standards.
- Facilitating Collaboration – Encourages consistent data use across the organization.
Now that you know what Data Governance is – let’s get to know how technically it is implemented to make things work!
Steps to implement AI Data Governance using best practices
A few technical steps can be performed to help in data management and governance, before feeding it to any AI tool or processing algorithms.
The implementation of these will ensure the trained AI data remains unbiased and accurate for the best results, enhancing the AI tool’s accuracy.
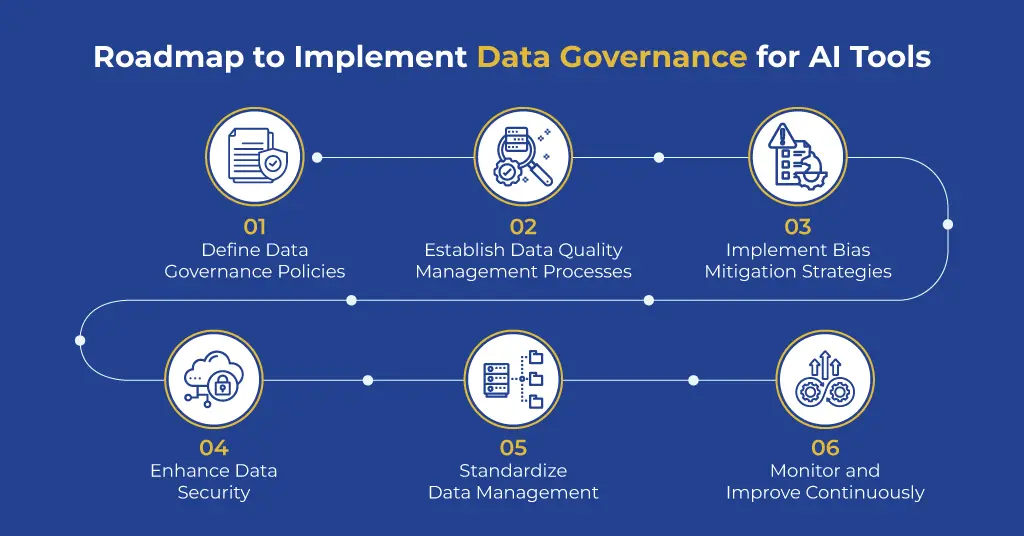
Step 1 - Define Data Governance Policies
- Create Standards – Develop and document data quality standards, including accuracy, completeness, and consistency metrics. These standards should align with industry best practices and regulatory requirements.
- Assign Roles – Establish a data governance team including data stewards, data owners, and data custodians who are responsible for implementing and maintaining these standards.
Step 2 - Establish Data Quality Management Processes
- Data Validation – Implement automated validation rules to check data accuracy and integrity as it is ingested into the system. This may involve using data profiling tools to assess data quality.
- Data Cleaning – Use data cleaning tools and ETL (Extract, Transform, Load) processes to correct or remove inaccuracies, duplicates, and inconsistencies. Data wrangling techniques are often employed here.
Step 3 - Implement Bias Mitigation Strategies
- Bias Audits – Employ statistical analysis and machine learning techniques to detect and quantify biases in datasets. Tools and frameworks like Fairness Indicators or IBM’s AI Fairness 360 can assist in this process.
- Diverse Datasets – Ensure that datasets include diverse and representative samples. This may involve sourcing data from multiple channels and conducting data augmentation.
Step 4 - Enhance Data Security
- Access Controls – Implement role-based access controls (RBAC) and encryption methods to protect sensitive data from unauthorized access and breaches. Tools like data loss prevention (DLP) systems and identity and access management (IAM) solutions are used.
- Compliance Checks – Regularly audit data handling practices against regulatory standards (e.g., GDPR, CCPA) using compliance management tools.
Step 5 - Standardize Data Management
- Uniform Formats – Define and enforce data formats, naming conventions, and metadata standards to ensure consistency across different data sources. Data modeling tools and schema management practices are utilized here.
- Integration Practices – Use data integration platforms and middleware to ensure seamless data flow and interoperability between different systems. Techniques like data federation and API management are commonly applied.
Step 6 - Monitor and Improve Continuously
- Performance Tracking – Use monitoring tools and dashboards to track the performance of AI models in real time. Techniques like model drift detection and performance metrics analysis help identify issues.
- Feedback Mechanisms – Establish feedback loops where performance data is analyzed to continuously refine data governance practices. This involves updating data quality rules and retraining models based on new insights.
By implementing these technical processes, organizations can ensure that their data governance framework supports accurate and reliable AI model training and operation, leading to better AI performance and outcomes.
Having a reliable team of data engineers aware of such data standards and practices can make a huge difference here!
Also Read;
But there is also something that needs to be taken care of!
As much as the data and its accuracy matter, below are some of the aspects that impact AI search results.
- AI performance also relies on the choice of algorithms and tuning of settings, not just data quality.
- Issues in the data, like errors or gaps, can still impact the model’s results.
- Models need regular updates to adapt to new data and changing conditions.
Considering these along with a Governed database drives the results we see.
By taking care of all these factors – there is a hope that in future we all can get access to AI that provides us with valid and precise data that is needed.
We live in a world where sometimes having no data is fine – but having inaccurate or biased data can create much more trouble than is anticipated!
Conclusion
It’s clear by now that with the rise of AI, data Governance is more important than ever!
We hear a lot about AI’s future and its potential issues, but what often gets missed is – How AI is trained.
It’s not just about having data anymore – it’s about the quality of that data and how carefully it is used.
We cannot ignore that even with all the technology, a lot of these processes are still controlled by humans.
How we handle and prepare the data can make a huge difference in the results we get.
By sticking to strong data quality governance practices and the strategies that are becoming more common, we can make sure AI reaches its full potential and truly delivers value.
Want to engineer your business data for an AI tool?
Let our experts take care of:
- Centralizing your business data from different software’s
- Help you analyze the data with custom dashboards
- Extract what’s important.
With our varied Data Engineering Services, we can help you achieve your data goals.
Schedule a one-on-one call to discuss your project requirements.